The Current Norm and its Limitations
Today’s AI landscape is dominated by a race to build ever-larger language models capable of a vast array of tasks. This trend is driven by the ambition to create a truly general artificial intelligence. While such models are undeniably impressive, they are often overkill for real-world business applications.
Domain Specificity Over Generalization
In reality, most AI use cases demand depth and specificity, not breadth. While generalized AI excels at generating novel ideas, it struggles with tasks requiring deep expertise in a particular domain. Financial analysis, legal research, and medical diagnostics all benefit from specialized AI models trained on a specific dataset.
The Benefits of Small(ish) Language Models
Small language models (SLMs) and small generative AI offer a compelling solution for domain-specific AI needs. They are easier to train, deploy, and maintain, making them a cost-effective option for businesses. Their smaller size also makes them more interpretable and easier to fine-tune for specific tasks, further enhancing their utility in real-world applications.
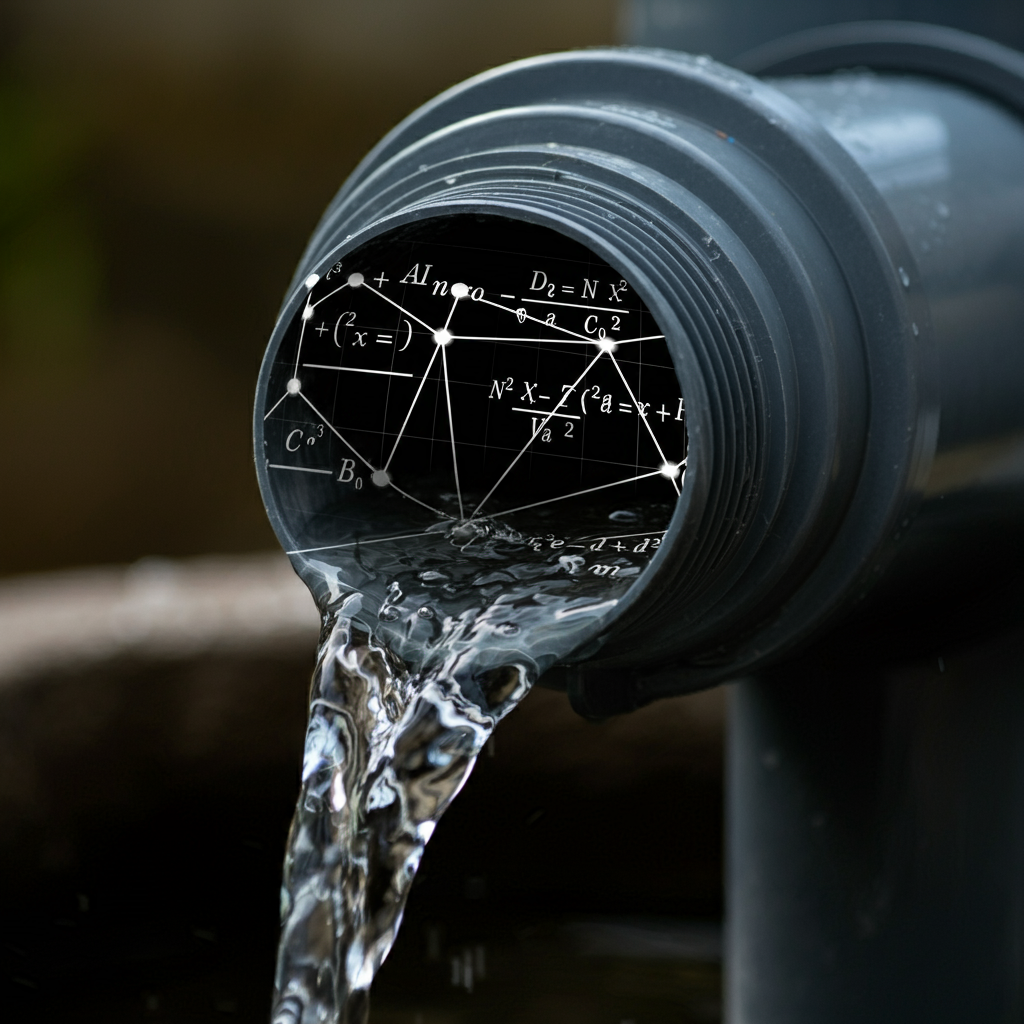
Grounding and Bounded Systems
Domain specificity aligns well with the concept of ‘grounding’ – ensuring AI models behave within clearly defined boundaries and constraints. This is easier to achieve with smaller, focused models than with massive, generalized AI systems. They are also more cost-conscious!
Ethical AI Through Design
The challenge of building ethical AI stems partly from the fear of uncontrolled, super-intelligent AI. Focusing on domain-specific AI mitigates this risk by design. Specialized models are less likely to ‘go off the rails’ because they lack the generalized knowledge and capabilities needed for autonomous action.
Technicity's Groundling Methodology and Implementation Framework
Technicity LLC’s Groundling Methodology and Implementation Framework provides enterprises with a structured approach to building grounded, domain-specific AI systems. This framework helps organizations define clear boundaries and constraints for their AI models, ensuring they remain focused, interpretable, and aligned with ethical considerations. By leveraging this framework, enterprises can confidently embrace the power of AI while minimizing the risks associated with unconstrained, generalized models.

Why This Matters
By embracing domain specificity and leveraging small language models, we can build AI systems that are not only more effective but also more ethically sound. It’s time to shift the focus from building bigger AI to building better AI – AI that is tailored to specific needs, transparent in its workings, and constrained by design.
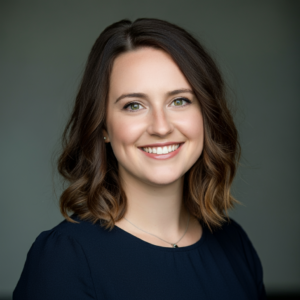
Aileen Sorio
GenAI Evangelist @ Technicity